MSc Student (Thesis: From Lab to Industry – Active Learning for Chemical Data / max. 83 hours per month)
Kennziffer: 2025-0110
- Frankfurt
- Part-time
- DKTK partner site Frankfurt/Mainz - Machine Learning/Bioinformatics in Oncology
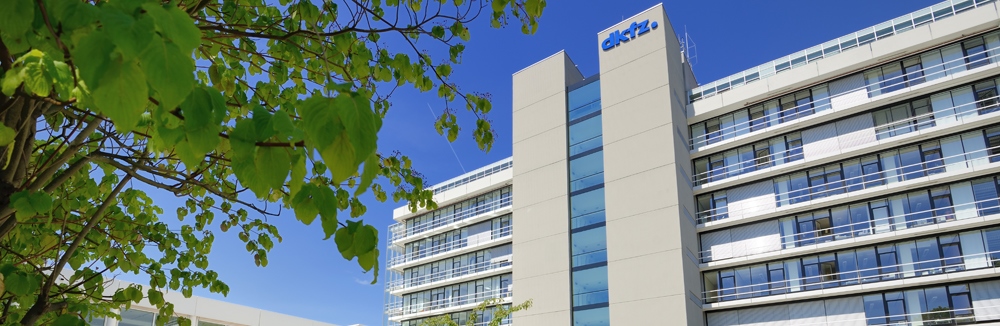
„Forschen für ein Leben ohne Krebs“ – das ist unsere Aufgabe am Deutschen Krebsforschungszentrum. Wir erforschen, wie Krebs entsteht, erfassen Krebsrisikofaktoren und suchen nach neuen Strategien, die verhindern, dass Menschen an Krebs erkranken. Wir entwickeln neue Methoden, mit denen Tumore präziser diagnostiziert und Krebspatient:innen erfolgreicher behandelt werden können. Jeder Beitrag zählt – ob in der Forschung, in der Administration oder der Infrastruktur. Das macht unsere tägliche Arbeit so bedeutungsvoll und spannend.
Together with university partners at seven renowned partner sites, we have established the German Cancer Consortium (DKTK).
For the DKTK Partner Site Frankfurt we are looking for as soon as possible a
We are seeking an exceptional MSc student to join our research group for “Machine Learning in Oncology” at the German Cancer Research Center (DKFZ) and Goethe University Frankfurt for an MSc thesis in collaboration with Bayer AG. The Buettner lab (https://mlo-lab.github.io) works on the intersection of machine learning and oncology, publishing original research in both areas at top-tier conferences and journals (e.g. Nature, NeurIPS, or ICML).
Relevant Publications:
Serra G, Werner B & Buettner F, How to Leverage Predictive Uncertainty Estimates for Reducing Catastrophic Forgetting in Online Continual Learning, Transaction on Machine Learning Research, 2025.
Serra G & Buettner F, Federated Continual Learning Goes Online: Leveraging Uncertainty for Modality-Agnostic Class-Incremental Learning, 2024, arXiv preprint arXiv:2405.18925.
Gruber SG & Buettner F, Uncertainty estimates of predictions via a general bias-variance decomposition. In International Conference on Artificial Intelligence and Statistics (AISTATS) 2023 (pp. 11331–11354). PMLR.
Hekler A, Brinker T & Buettner F, Test Time Augmentation Meets Post-hoc Calibration: Uncertainty Quantification under Real-World Conditions, AAAI Conference on Artificial Intelligence (AAAI) 2023
Gruber S & Buettner F. Better Uncertainty Calibration via Proper Scores for Classification and Beyond, Neural Information Processing Systems (NeurIPS), 2022
Your Tasks:
As a team member of the Buettner lab (https://mlo-lab.github.io), you’ll have the opportunity to research at the intersection of machine learning and chemistry. Through our partnership with Bayer AG, you’ll tackle real-world challenges using state-of-the-art machine learning approaches. This hands-on industry experience is powered by our lab’s theoretical work in uncertainty quantification. Building upon our established methodologies in uncertainty-aware predictive analysis, you’ll have the opportunity to drive innovation in this exciting field.
Join us for an exciting collaborative project investigating how different types of model uncertainty influence active learning in chemical applications. Your MSc thesis will focus on building and applying an active learning framework explicity leveraging decompositions of model uncertainty into aleatoric and epistemic components. Working closely with Bayer AG, you’ll see your theoretical advances translated into practical solutions for real chemical data challenges.
Your Profile:
Current enrollment in a Master's program in Computer Science, Statistics, Applied Mathematics, or related field at a German university. A good knowledge of machine learning methods and statistics is essential; familiarity with probabilistic modeling and uncertainty quantification is highly desirable. Very good knowledge of python and best practices in software development as well as experience with Linux environments are required.
The candidate will closely interact with other researchers, therefore good English communication skills are also required.
To apply please submit a single PDF file containing the cover letter, curriculum vitae and transcripts of records.
Unser Angebot:
- Hervorragende Rahmenbedingungen: modernste state-of-the-art Infrastruktur und Möglichkeit zum internationalen Austausch auf Spitzenniveau
- Zugang zu internationalen Forschungs-Netzwerken
- Flexible Arbeitszeiten
- Möglichkeit zur mobilen Arbeit und Teilzeitarbeit
- Entfalten Sie Ihr volles Potenzial: gezielte Angebote für Ihre persönliche Entwicklung fördern Ihre Talente
- Unser betriebliches Gesundheitsmanagement bietet ein ganzheitliches Angebot für Ihr Wohlbefinden